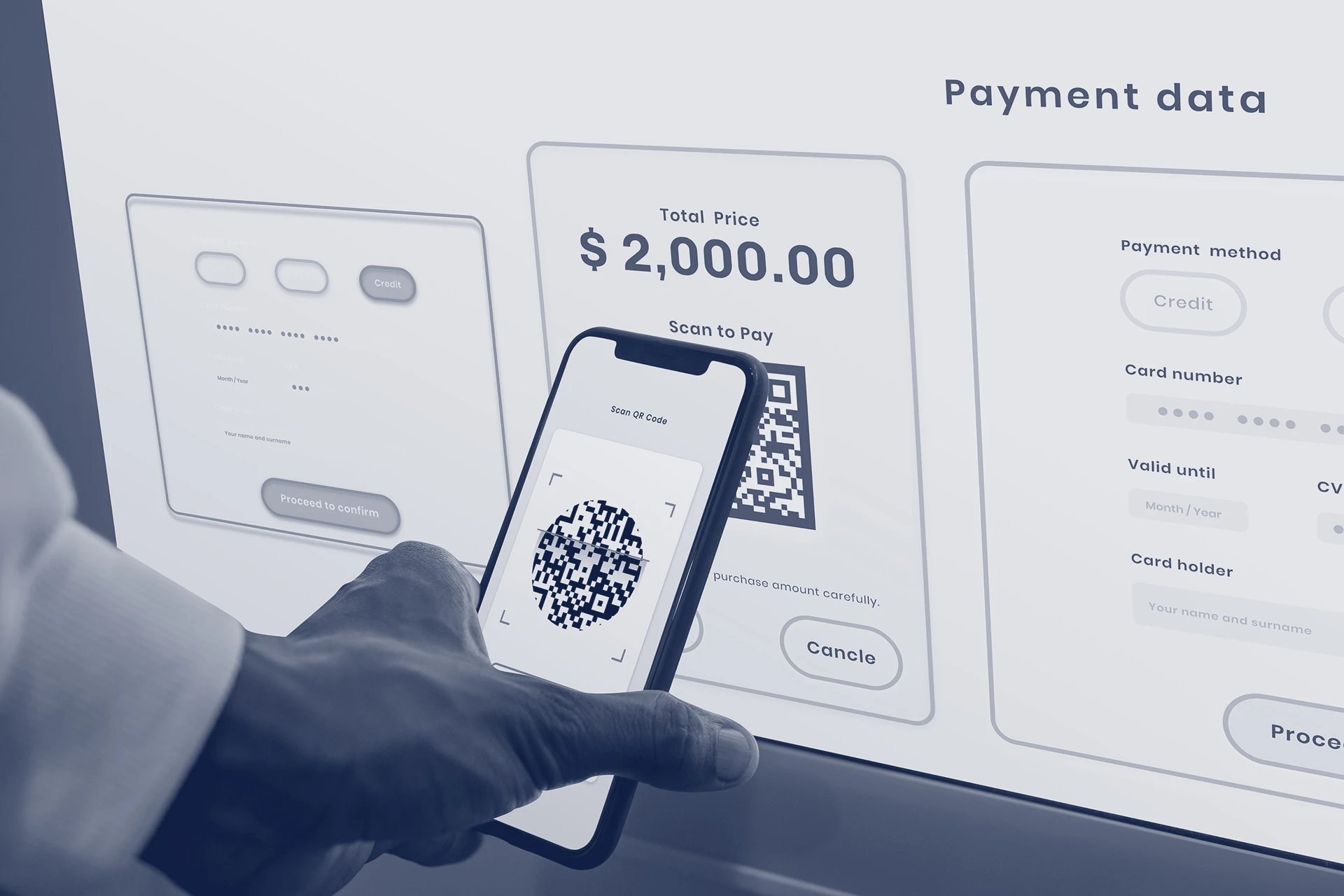
AI Billing in the Credit Era: How to Avoid the Cost Traps of Modern Usage Models
AI adoption is reshaping how we build software, but also how we price, consume, and bill for it. From generative models to inference APIs, the billing layer has become a strategic lever. But it's also become, in many cases, a hidden source of complexity.
As vendors race to productize AI capabilities, many are moving away from predictable pricing and into new territory: credit-based or token-based billing structures. These models promise flexibility. But without the right guardrails, they often introduce risk, confusion, and budget volatility.
In fact, according to a McKinsey survey, 85% of software companies plan to adjust their pricing models in the next two years, yet many lack the infrastructure to capture long-term benefits from these changes.
In this article, we’ll explore the evolving world of AI billing: how it’s changing, where the hidden cost traps are, and what product and procurement leaders can do to stay in control, while still unlocking the full value of modern AI solutions.
Understanding the Shifting Landscape of AI Billing
Gone are the days when AI billing was limited to simple monthly subscriptions or fixed usage tiers. Today’s AI billing models are far more dynamic, and more complex. We’re now seeing credits, tokens, provisioned throughput units, and metered usage that all vary based on function and volume.
Some vendors bundle AI features into broader product suites. Others offer AI services as standalone tools, priced purely on consumption-based AI billing. Either way, these newer structures often rely on metrics that are hard to benchmark or predict.
Why the shift? It comes down to how AI workloads behave. They’re unpredictable by nature—bursty, dynamic, and constantly changing. To match that unpredictability, vendors are adopting new billing formats, such as:
- Credit-based billing, where buyers pre-purchase flexible "units" that can be used across different services or features
- Token-based billing, commonly used in generative models, where output length or complexity determines cost
- AI usage billing, where charges are based on actual compute time, API calls, or other resource consumption
According to Gartner, by 2027, credit-based pricing will represent more than 25% of new spend with the top 10 enterprise software vendors. That signals a major shift that brings new challenges along with it.
Why Credit-Based AI Billing Can Be Risky
At first glance, credit-based AI billing sounds like a win. You commit to a pool of flexible credits and use them however you like across different services. But without transparency, that flexibility can quickly turn into a liability.
Too often, customers don’t have a clear picture of how credits are being used, or how much a specific task will actually cost. Multipliers can change across services. Credits might expire at the end of the term. And overages? They can be painfully expensive.
Here are some of the most common pitfalls:
- Lack of transparency. It’s not always clear how many credits a task will consume, especially when multipliers vary by service or usage type.
- No rollover. If you don’t use your credits by the end of the contract period, they’re often gone for good.
- Overages without protection. Go beyond your credit pool, and you could face rates that are 25–50% higher than your base pricing.
- One-way flexibility. Vendors often reserve the right to change credit consumption rates mid-contract, meaning your credits suddenly stretch less without any warning.
These risks are often buried in fine print or hidden behind generic contract terms. As usage increases and AI capabilities expand, these billing structures become harder to manage, particularly for finance and procurement teams tasked with budget accountability.
Real-World Examples That Highlight the Gaps
Let’s take a closer look at how some major vendors have implemented credit-based or usage-based AI billing – and where the cracks start to show.
1. Salesforce’s Einstein 1 Platform: Bundled but bounded
Salesforce includes a fixed amount of generative AI usage in its Sales GPT and Service GPT products. Sounds generous, right? The catch is that these credits are capped per user, and any overages are charged separately. If teams exceed the included usage, they must purchase additional blocks, often with little warning.
What’s missing is real-time visibility. Procurement teams often don’t know they’re approaching the threshold until the invoice arrives, and by then, it’s too late to adjust course.
2. Microsoft Azure OpenAI: Throughput commitments without clarity
Azure offers provisioned throughput units (PTUs) for accessing OpenAI services. In theory, this gives customers predictable access by reserving capacity. In practice? It’s tough to estimate how many PTUs you’ll actually need.
The lack of forecasting tools makes it easy to overcommit or under-provision. Either way, costs stack up. Worse, PTUs don’t guarantee delivery speed if demand spikes above what’s been reserved.
3. Elastic Cloud’s ECUs: Usage tracking done right
Elastic takes a more transparent approach. Their Elastic Consumption Units (ECUs) can be spent across a range of services, such as search, logs, metrics, and security. What sets them apart is their investment in clear tooling: usage calculators, real-time dashboards, and projections help customers understand exactly how resources are consumed.
It’s still a form of token-based billing, but with visibility built in. That transparency builds trust and gives buyers more confidence in their budgets.
Billing Models Shape Customer Trust and Retention
Your AI billing model directly influences how customers experience your product. When usage costs are unpredictable, hard to track, or shift without notice, trust erodes fast. And once that happens, you’re not just facing operational headaches. You’re looking at stalled expansion, churn risk, and a harder path to renewal.
A good billing experience, on the other hand, does the opposite. It builds confidence. It encourages experimentation. It gives finance and procurement leaders the clarity they need to budget, invest, and scale responsibly.
In fact, a recent industry analysis found that 60% of customers cite unexpected pricing complexity as a major barrier to scaling AI initiatives. That’s not just a procurement problem, it’s a severe growth blocker.
That’s why transparency, flexibility, and education are now essential features of any serious AI billing strategy. Vendors who invest in real-time usage dashboards, proactive alerts, straightforward contracts, and responsive support will stand out. Those who don’t, on the other hand, risk being perceived as opaque or adversarial, especially when CFOs start tightening budgets.
How to Build a Resilient AI Billing Strategy
If you're on the buying side of an AI solution, whether you're a product leader, procurement officer, or CFO, your billing structure isn’t just a contract detail. It’s a strategic decision. And with modern AI billing models, the risks are real.
Here are five ways to protect your business from AI billing risks and build a stronger foundation:
- Understand the model in detail
Ask your vendor to break down exactly what a credit, token, or unit represents. Get service-by-service breakdowns, pricing formulas, and real-world usage examples written into the contract.
- Push for rollover and swap flexibility
If you’re prepaying for credits or tokens, you should be able to roll unused ones into the next term, or swap them between services. If that’s a no-go, negotiate a discount or reduce your commitment.
- Lock in multipliers or demand notice periods
Avoid contracts that allow vendors to tweak multipliers mid-term. If locking isn’t an option, push for a six- to twelve-month notice period and the right to reprice or exit if terms change.
- Clarify overage policies
Make sure your agreement includes a cap on overage rates, ideally no more than 10–15% above base pricing. And insist on usage alerts as you approach your threshold.
- Align internal stakeholders early
Finance, procurement, and product teams all need visibility into billing risks, contract flexibility, and usage assumptions. When these teams are aligned, it’s easier to plan ahead—and avoid surprises.
Billing Is Not Just a Back-Office Concern, It's Part of the Product Experience
In the AI era, billing isn't just a transactional back-end process. It's part of the product experience. It reflects how vendors treat their customers, how well they understand real-world usage patterns, and how committed they are to long-term success.
The most effective AI billing strategies combine two key ingredients: flexibility and predictability. They give customers room to experiment, without the fear of surprise charges. They make it easy to scale, without stepping into a budgeting black hole.
Vendors who get this right improve margins and win long-term loyalty.
At Vayu, we believe your billing model should be as intelligent as the systems it supports. That means pricing structures that adapt, inform, and empower—rather than restrict. Because in a world defined by AI-driven growth, the smartest billing strategy is the one that puts the customer first.
FAQ
1. What is credit-based AI billing and why is it becoming popular?
Credit-based AI billing allows users to pre-purchase flexible units (credits) that can be used across different AI services. It’s gaining traction because it offers flexibility in managing unpredictable AI workloads—but it also carries risks if credit usage lacks transparency or protections.
2. How do token-based billing models work in AI?
Token-based billing is commonly used in generative AI, where you're charged based on the number or complexity of tokens processed (i.e., words or code segments). This model directly links cost to usage but can be hard to forecast without the right tooling.
3. How can companies reduce AI billing risks?
To minimize risks, companies should demand clarity in contract terms, push for rollover and usage alerts, align internal stakeholders early, and ensure vendors provide visibility into how costs are calculated and tracked.