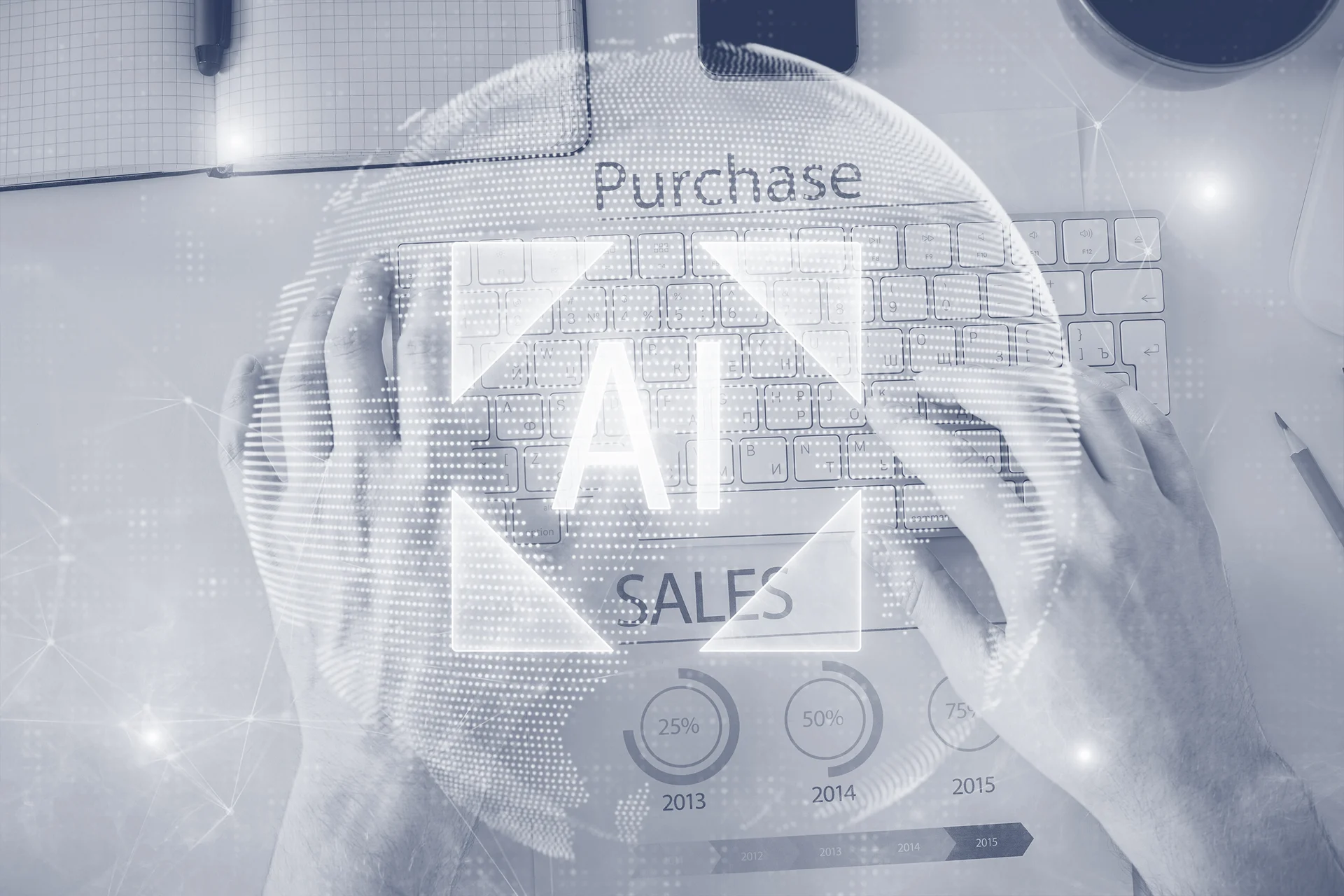
Pricing AI Agents: How to Price and Monetize Agentic AI
AI agents represent a new frontier in software—one that redefines how technology operates, how businesses function, and how value gets delivered. Instead of waiting for human input, these autonomous tools can think, decide, and act on their own. It's a shift in the very foundation of how we build and use software.
But with this shift comes a new challenge: How do you price something that thinks and acts on its own?
This isn’t a theoretical exercise. According to Gartner, by 2028, a third of enterprise software will include agentic AI—up from less than 1% today. By 2029, more than half of machine customers will operate with enough intelligence to make proactive decisions. And by then, at least 15% of all day-to-day work decisions will be made autonomously by agents.
We’re not talking about incremental change. We’re talking about a complete rewrite of the software playbook.
For product leaders, CFOs, and anyone thinking about monetization, this raises urgent questions. Traditional SaaS pricing models—like charging per seat, per user, or per feature—don’t fit this new world. And the market? It’s learning that the hard way.
Why Pricing AI Agents Is So Different
AI agents don’t fit into the pricing frameworks we’ve come to rely on. In the world of traditional SaaS, pricing usually scales in a straight line—per user, per seat, per API call. It’s predictable, measurable, and easy to map to usage. AI agents disrupt that equation entirely.
By design, these agents are dynamic and autonomous. They don’t just wait for a user to click a button—they make decisions, take action, and sometimes operate entirely on their own. One agent might complete hundreds of micro-tasks in a single day. Another might replace hours of repetitive human work with a few lines of code.
The value they generate is enormous—but it’s also harder to track. How do you price something that creates outcomes rather than just usage? And how do you tie your ai agent pricing strategy to impact, not just activity?
This is the heart of the challenge: we’re no longer just pricing software—we’re pricing autonomy, intelligence, and results. And that calls for a different way of thinking.
AI Pricing Models: Two Emerging Approaches to AI Agent Monetization
As AI agents gain traction, two distinct AI agents pricing models are starting to emerge. Each reflects a different philosophy—and a different level of appetite for risk. Each reflects a different philosophy—and a different level of appetite for risk. But both are shaping the future of how AI agents will be priced, packaged, and sold.
Approach 1: The Adoption-First, Monetize-Later Playbook
This approach prioritizes usage over immediate revenue. The goal is simple: get AI agents into users’ hands with as little friction as possible. That often means free tiers, bundled access, or low-commitment pilots.
The thinking is clear: the more users engage with the agent, the more they’ll discover its value. Once the product becomes essential, monetization can follow.
It’s a familiar playbook—mirroring early freemium models in SaaS, where growth came first and monetization came later. It’s especially useful when the product is still evolving, or when teams are still figuring out where customers find the most value.
The biggest upside? Data. Real usage patterns reveal what matters to users, which in turn helps shape a pricing model grounded in behavior—not assumptions.
The tradeoff, of course, is delayed revenue. Without a clear pricing structure, it’s harder for finance teams to forecast growth, assess CAC-to-LTV ratios, or scale GTM efforts confidently. Still, for many AI startups, the early learnings outweigh the short-term financial ambiguity.
Approach 2: Monetize from Day One with Value-Based Pricing
The second strategy takes a bolder stance: charge early and price for impact.
Companies in this camp believe their agents deliver immediate, measurable value—and they price accordingly. Instead of waiting to optimize monetization, they launch with premium, value-based pricing from the start.
This might mean usage-based billing, per-action pricing, or even outcome-based models tied to business metrics like resolution rates, productivity gains, or revenue impact. The common thread: pricing that reflects performance, not just features.
It’s a strong message to the market: this isn’t a side experiment—it’s a tool that drives real results.
But this approach demands confidence. If the agent underperforms—or if the customer can’t clearly connect the dots between usage and value—it can create friction, especially among early adopters who are still exploring.
We’re already seeing this model gain momentum with established players like Salesforce and Zendesk, both of whom are actively redefining how AI agents are priced at scale.
Salesforce: Betting Big on AgentForce
Salesforce has never been one to play it safe—and its AI pricing strategy reflects that. With AgentForce, part of the broader AI Cloud suite, the company is making a bold move: $360 per user, per month.
That’s not a lightweight fee—and it’s not meant to be.
What makes this approach stand out is how Salesforce frames the value. Instead of charging based on usage, tokens, or interactions, Salesforce is helping redefine how companies are pricing agents AI at scale—with a bold, flat-rate approach that reflects agent value, not activity.
The rationale? It’s not just a tool—it’s a digital co-worker. One that actively supports sales, service, and marketing teams by taking real, autonomous action.
The pricing reflects the expectation of meaningful productivity gains—and positions AgentForce as a high-impact contributor, not a background utility.
There’s clarity here, too. Customers know exactly what they’re paying, and what kind of business outcomes to expect. No metering. No guesswork.
But there’s a strategic message embedded in this model as well. By pricing AgentForce with confidence, Salesforce shifts the narrative from “what features does this agent have?” to “what results will it deliver?” It’s a strong move—and one that’s likely to influence how others think about pricing autonomous software.
Zendesk: Pricing Based on Real Outcomes
Zendesk is taking a different—but equally strategic—path when it comes to monetizing AI agents. Instead of seat based pricing, consumption based pricing, or bundling into traditional tiers, Zendesk has introduced outcome-based pricing.
Here’s how it works: customers are charged based on the number of customer support tickets successfully resolved by the AI agent—autonomously. If the customer service agent doesn’t deliver a resolution, there’s no charge.
It’s a simple, powerful value proposition: you only pay when the agent performs.
This model tightly aligns incentives. Zendesk is motivated to ensure the agent performs at a high level—because their revenue depends on it. Customers, meanwhile, avoid paying for failed interactions, partial responses, or bloated usage metrics. They’re paying for actual, measurable business outcomes.
Of course, this approach requires more operational discipline. Outcome attribution must be crystal clear. Customers need to trust the metrics, and Zendesk must deliver consistent, high-quality results to justify the pricing.
Still, for enterprise buyers looking to minimize risk and maximize ROI, it’s a compelling proposition. The agent becomes more than a tool—it becomes a performance-driven partner. And that’s a fundamentally different way to think about software and AI models.
Common Pricing Models Taking Shape
Beyond Salesforce and Zendesk, we’re seeing a variety of pricing models take shape across the AI agent landscape. While no single standard has emerged yet, a few common approaches are gaining traction:
- Per-task or per-action pricing, where customers are billed based on the number of tasks an agent completes.
- Usage-based pricing, which ties cost to metrics like data processed, time used, or number of API calls.
- Tiered pricing, offering access to different levels of agent intelligence or capabilities at different price points.
Then there are hybrid models—combinations of flat-rate subscriptions with usage- or outcome-based components. These give vendors predictable baseline revenue, while still allowing them to capture value when agents perform exceptionally well.
The common thread across all of these? Pricing is moving away from rigid product tiers and toward more flexible, performance-aligned models. These flexible models better reflect how customers experience value in AI-driven environments, where agents act autonomously and outcomes matter most.
Pricing AI Agents is Not Just a Product Call
Traditionally, pricing decisions lived between product marketing and sales operations. But with AI agents, that responsibility now spans far beyond those boundaries. Pricing has become a strategic lever—one that touches finance, engineering, GTM teams, and customer success.
And that creates a new kind of operational challenge.
Most companies still rely heavily on engineering teams to implement pricing changes. Updating a tier, testing a usage-based model, or launching an outcome-based experiment can take weeks—sometimes months—of dev time and data analysis.
In a space where AI capabilities are evolving by the week, that kind of lag is a real liability. The ability to quickly adapt pricing models to match product performance and customer value is becoming a competitive advantage.
CFOs Need to Own Pricing Agility
For CFOs, the rise of AI agents introduces both risk and opportunity. Static pricing models, long implementation cycles, and limited room for experimentation simply won’t cut it anymore. To stay competitive, CFOs must take a more proactive role in shaping and evolving pricing strategy.
This starts with having the right tools—systems that enable pricing autonomy. CFOs need the ability to launch new models, test them in-market, and adjust quickly based on real-world performance.
It also requires visibility. That means real-time insights into how pricing changes are impacting revenue, retention, usage patterns, and overall customer behavior.
But perhaps most importantly, it demands a shift in mindset. In the AI-powered economy, CFOs aren’t just financial stewards—they’re growth leaders. Their decisions around pricing will
increasingly shape how value is captured, scaled, and reinvested.
Final Thoughts: Pricing Is the Next Strategic Advantage
AI agents aren’t just changing how products are built—they’re redefining how value is delivered, experienced, and monetized. This isn’t just a product shift. It’s a business model transformation.
Companies that treat pricing as an afterthought will struggle to keep up. But those that approach it as a strategic capability—adaptive, data-driven, and outcome-focused—will shape customer expectations, influence buying behavior, and gain meaningful competitive advantage.
Salesforce and Zendesk have already demonstrated what’s possible when pricing aligns with performance and impact. The next wave of innovation won’t just come from smarter agents—it will come from smarter monetization strategies.
For CFOs and executive teams, the mandate is clear: own pricing agility. Move fast. Experiment often. Build systems that support dynamic pricing at scale.
Because in the era of autonomous software, pricing isn’t just a revenue lever—it’s a relevance lever.
FAQ
What makes pricing AI agents different from traditional SaaS pricing?
AI agents operate autonomously and deliver outcomes, not just usage. Traditional SaaS pricing models—like per seat or per feature—don’t capture the value created by agents that think, decide, and act on their own. Pricing needs to reflect performance and impact, not just activity.
How are companies like Salesforce and Zendesk pricing their AI agents?
Salesforce uses a bold flat-rate pricing model ($360/user/month) to reflect the value of AgentForce as a digital co-worker. Zendesk charges based on outcomes—specifically, the number of customer support tickets resolved autonomously by the AI agent.
Why should CFOs be involved in AI pricing strategy?
AI agent pricing requires agility. This is why CFOs need to be involved in implementing flexible, testable pricing models that evolve with customer behavior and product performance. In the AI economy, pricing is an important strategic growth lever.